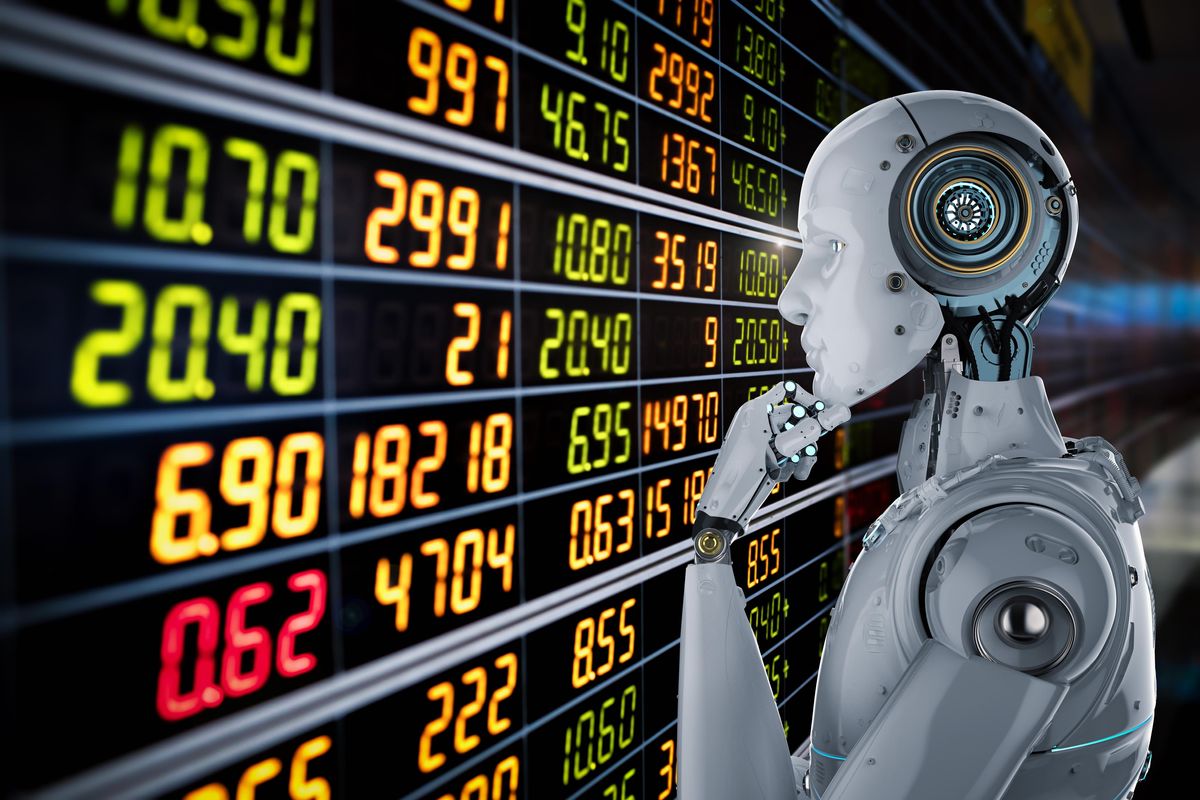
INN spoke to Rick Roche of Little Harbour Advisors about risk modeling, natural language processing and alternative data sets in investing.
Artificial intelligence (AI) has the potential to massively impact investment firms, analysts, investors and the overall wealth management industry.
Through their use of vast data sets, extending everywhere from satellite technology and sensors to consumer and macroeconomic data, AI and machine learning have the ability to shift investors’ views on traditional financial data. AI has the potential to generate considerable competitive advantages and inform investment decisions.
According to a 2017 report from JPMorgan (NYSE:JPM), machine learning will have an impact on both quantitative and fundamental investors. A number of companies are integrating predictive analytics, learning algorithms, AI solutions, expert systems and numerous data points into their systems.
For example, there is sentiment analysis firm Social Alpha, whose former CEO, Prem Melville, worked on IBM Watson. The firm is suited for the quantitative and fundamental investing camps, the report notes. Social Alpha provides clients with both market sentiment indicators and volatility indicators.
Rick Roche, managing director of Little Harbour Advisors, spoke to the Investing News Network (INN) about AI’s integration into the investment management sector.
Roche discussed AI uses, such as non-traditional data sources, relevance determination and macroeconomic analysis, risk modeling and natural language processing (NLP).
AI algorithms are being coalesced with human intelligence for investment managers and actors in the financial services industry. Fund managers and private equity firms are applying AI analytics to their portfolio decision making, management processes and future risk perspectives.
Here’s a look at three primary applications of AI and machine learning for investing, according to Roche.
1. Non-traditional data sources
With the relatively recent availability of mountains of data, AI has been applied to gather alternative data sets to help find alpha (the performance of a holding in relation to the market) to achieve higher returns.
“Alternative investment data are sources of data that might come from satellites, or GPS, company exhaust, which could be an anonymous survey from employees or customers, credit card receipts, emailed receipts — they’re all kinds of alternative investment data,” said Roche.
As many investors have access to the same traditional data, non-traditional data sets and indicators can serve as key differentiators, especially if they are based on real-time data.
2. Natural language processing
As a subset of alternative data sets, NLP can be used to indicate sentiment, and then can be further applied to determine information relevancy and macroeconomic analysis.
“In fact, one of the powers of NLP, which has been around since the 1940s, I believe, is that it’s much more powerful in terms of deciphering sentiment,“ said Roche.
Computational linguistics pioneer Roberto Busa was among the first to use NLP in the 1940s. In 1949, he met with Thomas J. Watson, founder of IBM (NYSE:IBM), to ask that Watson sponsor his compilation of Thomas Aquinas work that was read by a computer. It took 30 years to complete the process. NLP began to gain more momentum during the 1950s when it was being used for translation purposes.
Sentiment analysis is an application of AI and NLP that can determine if a body of text has a certain effect — whether it is positive or negative, for example.
NLP is a form of AI technology that analyzes and aims to understand human language.
Roche detailed two formative players applying NLP in the investment space: Prattle and RavenPack.
Prattle, founded in 2014 by Harvard graduate Evan Schnidman, is based in St. Louis. Prattle helps determine sentiment through being fed 5 to 6 million document pages a day. In turn, the NLP system determines whether sentiment on a company is bullish, bearish or neutral.
RavenPack, a pioneer in the NLP space, has been in operation since 1998. Through NLP, RavenPack reads all of the news and press releases from the Dow Jones Industrial Average (INDEXDJX:.DJI) newswire. In a matter of seconds it rates and determines each news piece’s relevance to a given company, Roche said. If a company is deemed relevant, it is then given a sentiment analysis score.
When applying NLP to macroeconomic analysis, NLP programs have the ability to use multiple data sets and indicators. According to JPMorgan, there are 277 macroeconomic data indicators, including currency prices, commodity prices and market prices foreign exchange rates.
Schnidman wrote his dissertation on the application of NLP in analyzing US Federal Reserve decisions.
“What Prattle did first was follow the 15 largest central banks in the world and basically read everything, any kind of transcript, any kind of document, any kind of audio recording,” said Roche.
Roche further noted that Prattle was among the few to accurately anticipate, against consensus, that the Bank of England would not lower rates after Brexit in June 2016.
The macroeconomic analysis features of NLP attract large, global macro hedge funds.
“One of the biggest buyers of Prattle’s product were hedge funds that were discretionary global macro managers,” Roche said. “They wanted to have a sense of what’s happening in international markets, what’s happening with currencies, with governmental changes, with regulatory changes.”
3. Risk modeling
The application of AI systems to risk modeling has the potential to provide a more extensive, nonlinear and robust model, noted Roche.
AI applications stand in contrast to the majority of existing risk models, which apply linear and normal distribution assumptions, he added. In contrast, the application of AI into risk models has the ability to combine macroeconomic data and predictive analysis to the risk model’s framework.
In addition, AI can be applied to position sizing and asset allocation in asset management. According to a paper published in the International Journal on Advances in Intelligent Systems, asset allocation methods have been studied through applied neural networks to analyze historical price data, macroeconomic factors and the corresponding stock movement.
The study found that the performance of AI-driven models was superior to a passive investment strategy between the time period of February 2008 and August 2017.
Investor takeaway
A number of hedge funds and private equity firms are applying AI strategies. These firms include Renaissance Technologies, Two Sigma, Bridgewater Associates and DE Shaw Group. Renaissance Technologies, a forerunner in applying AI to investment management, realized 35 percent in average annualized gains between 1990 and mid-2018. Firms are synthesizing human analysis with advanced statistical algorithms to improve performance and gain greater insight on the market.
In addition, portfolio management companies based in Canada are also offering services that integrate AI and deep learning, including Investifai and Castle Ridge Asset Management. For a more comprehensive overview of funds that are applying these methods, click here.
Don’t forget to follow us @INN_Technology for real-time news updates!
Securities Disclosure: I, Dorothy Neufeld, hold no direct investment interest in any company mentioned in this article.
Editorial Disclosure: The Investing News Network does not guarantee the accuracy or thoroughness of the information reported in the interviews it conducts. The opinions expressed in these interviews do not reflect the opinions of the Investing News Network and do not constitute investment advice. All readers are encouraged to perform their own due diligence.