How Big Tech is Helping Leverage Big Data in Healthcare
Industry leaders shared their thoughts on how emerging technologies like AI and machine learning can help the healthcare industry advance.
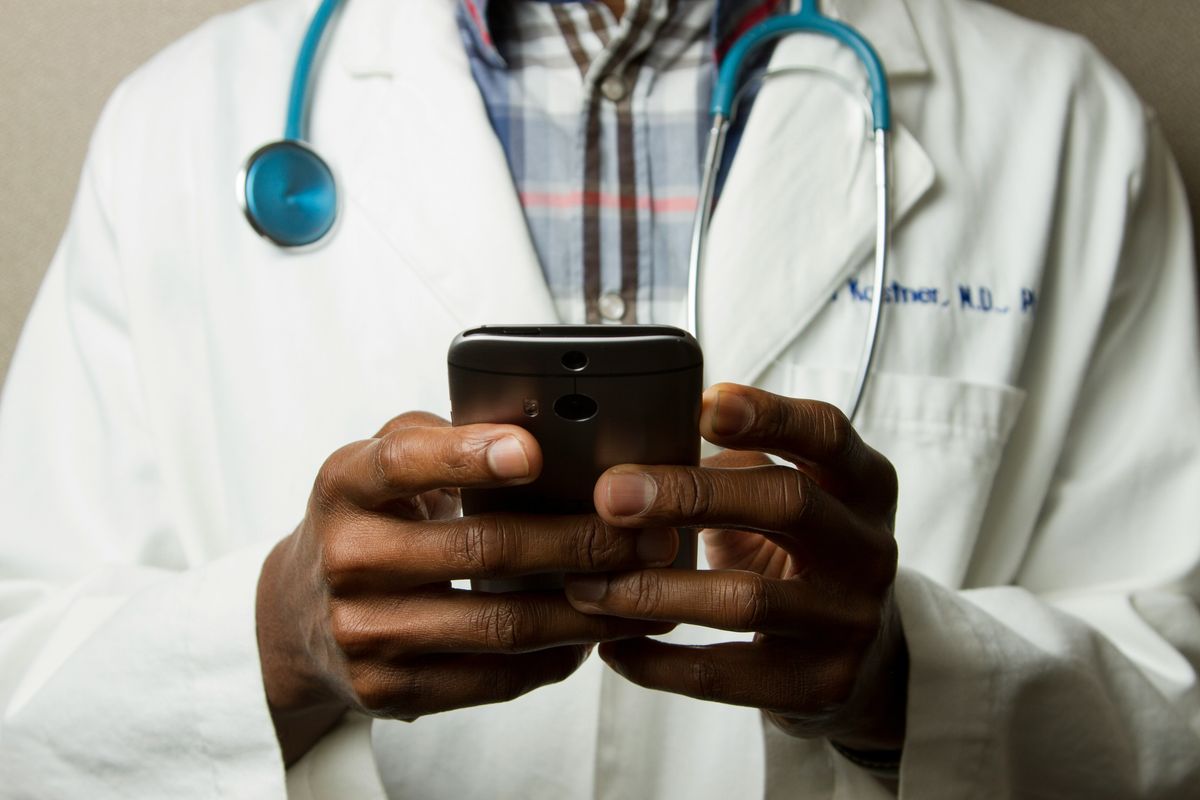
The use of artificial intelligence (AI) and machine learning in healthcare was a prominent theme discussed at this year's Biotech Showcase, a three day event held in San Francisco in January.
While experts agree that Big Tech has the potential to improve patient outcomes by revolutionizing the way illnesses are diagnosed and treated, there are also challenges and ethical considerations when implementing AI in healthcare.
The Investing News Network sat in on several Biotech Showcase discussions on the role of AI and machine learning in healthcare, as well as the impact of digital medicine. These talks shed light on the opportunities and challenges of bringing these industries together, and explored strategies for overcoming obstacles and maximizing the benefits of these technologies. Read on to learn about key takeaways from the event.
How are AI and machine learning being used in healthcare?
One of the livelier discussions during the Biotech Showcase took place on January 9, when experts in the field gathered to discuss the challenges and opportunities of integrating AI and machine learning in healthcare.
During a panel, participants referenced recent deals made between Isomorphic Labs, Alphabet's (NASDAQ:GOOGL) digital biotech company, and Eli Lilly and Company (NYSE:LLY) and Novartis (NYSE:NVS), describing them as a signal that Big Tech players are ready to expand their reach in the healthcare sector.
“The top segments dominating AI today are robotic-assisted surgery, clinical trials, content machines, drug dosage error, diagnostics and drug discovery. The top driver in growth is the amount of medical data. According to Datacon, the healthcare data explosion will exceed 10 trillion gigabytes by 2025. This is a significant growth in the amount of data that humans wouldn't be able to mine at all. We need these machines to help mine it,” said moderator Beth Rogozinski of Oncoustics before introducing the panel. She pointed out that global issues like pandemics and chronic illnesses are driving acceptance and adoption of AI as a more integral part of the healthcare ecosystem.
The topic of AI made its way into several other conversations over the three days of the Biotech Showcase. “More recently, there has been an effort alongside many startups and larger companies in our industry to look at how AI and these technologies can be used to enable discovery and bring more efficiency and cost-effectiveness and insights to the drug-discovery process. So I think that gives a little bit of a flavor for how we are using AI,” said Stacy Feld of Johnson & Johnson Innovation during a January 8 discussion on the landscape of partnering and business development.
However, as eager as Big Tech is to get involved in biotech, the business models are starkly different, as was pointed out by Atomwise's Abraham Heifets. “There's a fundamental mismatch in how you ascribe value,” he said.
Biotech companies focus on developing drugs using platform technologies that tend to become commoditized quickly, making it a challenge for them to maintain a competitive advantage for long periods. In contrast, tech companies leverage platform technologies with network effects that allow them to maintain dominance for decades.
Heifets alluded to a potential arbitrage opportunity if investors are confident in the long-term sustainability of AI-driven biotech companies' competitive edge. “So the question is, how do you combine these? How do you do the interface correctly when people are optimizing for fundamentally different goals?” he asked. In his view, the industry will need to explore different ways to combine the strengths of tech and biotech while navigating each sector's unique challenges.
And, as Heifets noted, while discussions of AI in drug discovery often focus on improving speed and reducing costs, the true value drivers are first-in-class and best-in-class drugs. Given that current AI models tend to generate similar answers, limiting their ability to discover unique and effective drugs, the challenge essentially lies in leveraging this technology to develop genuinely novel and transformative therapies.
“In my field, there's been a number of high-profile papers producing machine-learning models that predict new antibacterials, and then you go and look at the molecules that they're predicting, and it's ... very closely related molecules,” said Kurt Thorn of ArrePath. “Identifying novel material ... is hard, and I think one way to approach that is to tightly couple the machine learning with the experimentation so that you can explore chemical space and learn new things quickly and update your models with that. I think (that) will be an interesting challenge for some of these Big Tech partnerships, because it means you need to really closely integrate the machine-learning teams and the wet lab teams.” Innovation will come from a balance between AI and generative AI and human thoughtfulness and oversight.
Of course, there are limitations to what AI can achieve if it's not trained properly. As tech and pharma become more intertwined, the two sectors will have to come up with ways of working together to fully leverage the benefits of AI.
As Heifets told the Biotech Showcase audience, “On this side, we might have the solutions to drug discovery, which are discoverable by humans. And then over here, overlapping but not enclosed, might be the solutions which are discoverable by AI. If we do what's standard today, computer-aided drug discovery, you get the intersection of these two, what the software suggests that then the human will accept. And what we want if we're really going to have breakthroughs is not the intersection. It's the union of those two.”
Can data bridge the gap between healthcare and Big Tech?
So how can companies bridge the gap between the healthcare and tech sectors to achieve a union of human and machine solutions that can lead to real breakthroughs? Experts believe harnessing data may be crucial.
“I think the driving future in biotech, specifically where AI is involved, is in the generation and curation of datasets that can be used to train and empower all of the complex models around it. We're not really facing a bottleneck on the complexity of the models. We are facing a bottleneck in the complexity of the data and the applicability of the data to the outcomes that we want to infer through the models that we train,” said Andrei Georgescu of Vivodyne, reinforcing the idea that the increasing availability and quality of medical data are key factors in advancing AI’s capabilities in healthcare.
During another panel discussion, "The Impact of Digital Medicine Innovation," experts explored various strategies and technologies for collecting digital health data, such as wearable devices and mobile apps, which can be used to facilitate more data-driven and personalized approaches to healthcare and drug discovery.
“I've got a demo on my wrist that does blood pressure and another one that'll do blood sugar, and (I) expect in a couple of iterations the Apple (NASDAQ:AAPL) Watch will do real-time blood sugar and blood pressure and have this healthcare continuum of your digitome that eventually will plug into your clinician ... and they'll need to look at the raw data, look at the insights and the actionable information that they can use, or the patient can use themself in terms of self-care,” Daniel Kraft of Next MedHealth told attendees at the Biotech Showcase.
Chris Benko of Koneksa echoed this sentiment, saying, “I think that's where in the near future we'll be able to put all this data into insights for ... the patient in front of me, and not just base their clinical plan on some 10 year old, double-plan procedural, Framingham study, but something that's really relevant from a data lake that's real time and updated.”
The slow adoption of digital solutions in healthcare has been a major obstacle to unlocking the full potential of data-driven solutions. While other industries have embraced digital technologies at a rapid pace, healthcare has lagged behind, due in part to a variety of challenges on both the patient and provider side. As Christine Lemke of Evidation pointed out, most patients only use health-monitoring technology consistently when it’s offered as a "side effect."
For example, when a device is multi-functional, such as a Fitbit, most users aren’t using it for the health-monitoring features, they’re using it for other functions; in this case, to tell the time.
On the provider side, there can be resistance to integrating new digital tools due to a lack of time to learn about them, a lack of information about available options or a preference for traditional methods. A huge challenge facing the healthcare industry is the simultaneous requirement of training its workforce to adopt new technologies and redesigning training protocols to keep pace with rapid innovation. Susan Kirsh of Health for Discovery, Education and Affiliate Networks said that this is particularly challenging because the rate of technological innovation is not linear. This can slow down the adoption of digital solutions and limit the amount of data collected.
Another significant shift in the landscape of data collection is the move toward continuous data gathering. "(Continuous data) is very different than traditional clinical data that's spot checked. It's been relatively small. Having continuous measures over time — and having to even deal with that temporal element of things — is beyond the basic sort of biostatistics training that a lot of people who are tackling these problems are familiar with. It's a different kind of math problem (that's geared) more toward people with an informatics or a physics background and so forth,” said Benko.
“I think there's enormous potential of AI tools to help bridge that gap between the skillset of the person trying to deal with the data and the ability to manipulate and use it, but there also still has to be a significant upskilling, I think, of people that come in to work with these systems in data versus traditional clinical data sets. It really just is much denser, much more complex. It has many more elements," he added.
In terms of fair and representative data, experts mentioned the US Food and Drug Administration's push for inclusive datasets as a positive step toward reducing bias and ensuring that all populations are considered in the development of new therapies and technologies. The decentralized approach to data collection mentioned by Lemke has the potential to improve access to diverse populations and gather more comprehensive data.
However, monetizing this data and ensuring its widespread adoption remains a challenge, and as Kraft pointed out, the lack of clear incentives and funding mechanisms can hinder the scaling of digital health innovations. Collaboration between companies and alignment with payer incentives will be crucial for moving the adoption of new technologies forward and ensuring that they have a positive impact on patient care.
Investor takeaway
The Biotech Showcase discussions on how AI and machine learning are beginning to affect the healthcare sector highlight the opportunities and challenges of integrating Big Tech with biotech.
While AI has the potential to revolutionize drug discovery, current limitations are making it tough to develop genuinely unique and effective therapies. The industry needs to explore different strategies to leverage the benefits of AI while navigating each sector's unique challenges. The integration of machine learning and wet lab teams will be crucial to balancing the integration of AI and generative AI with human clinicians — ultimately, the collaboration between Big Tech and healthcare could lead to significant advances in the sector and improvements for patients.
Don’t forget to follow us @INN_LifeScience for real-time news updates!
Securities Disclosure: I, Meagen Seatter, hold no direct investment interest in any company mentioned in this article.